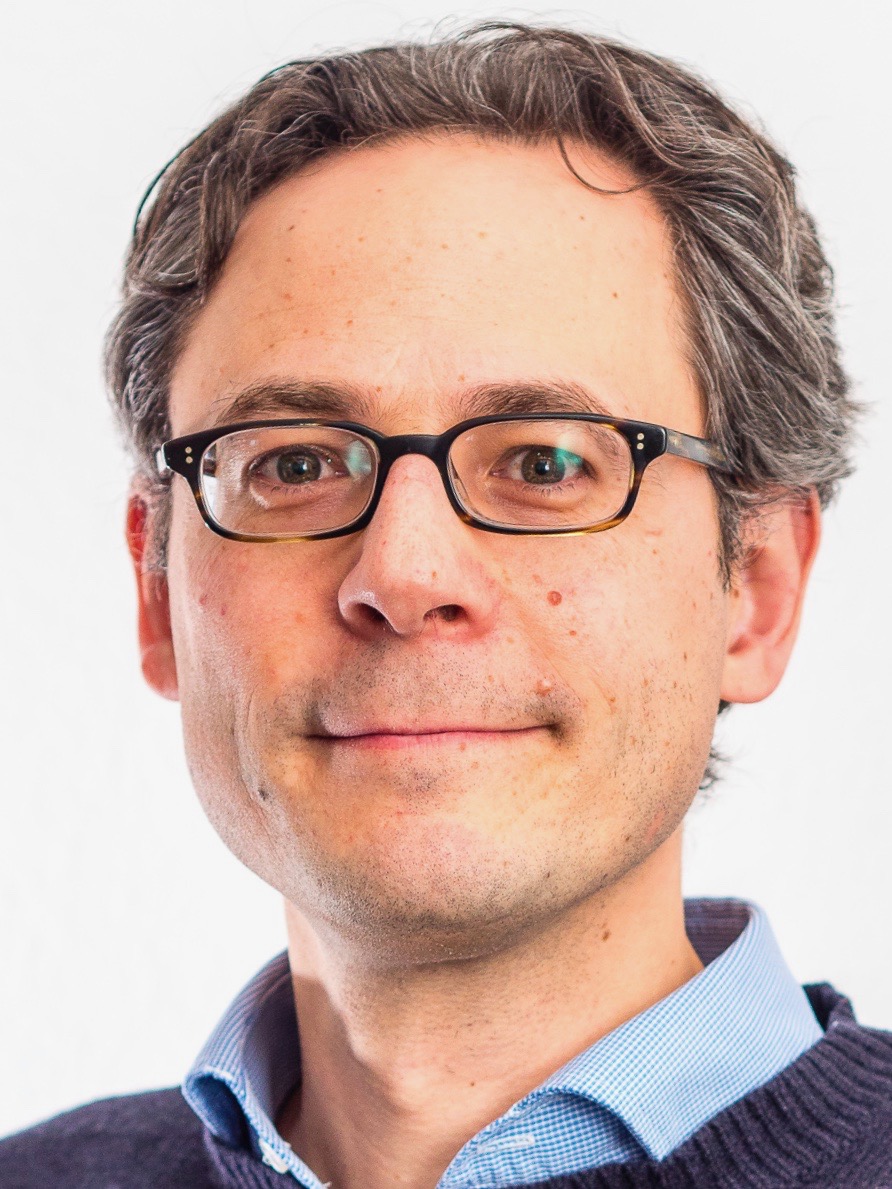
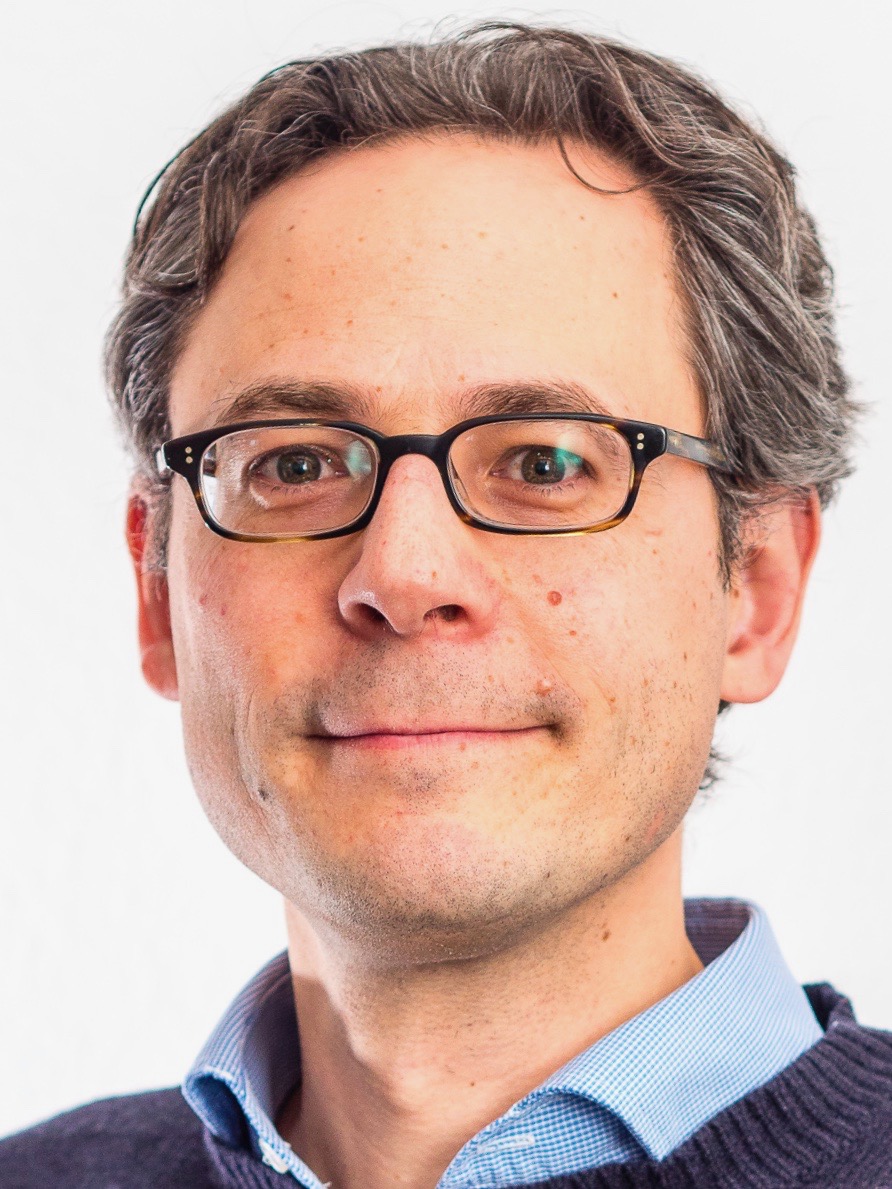
Topics in Mathematics Münster
T8: Random discrete structures and their limitsT10: Deep learning and surrogate methods
Research Interests
$\bullet$ Complex networks and non-standard growth models.
$\bullet$ Stochastic algorithms, numerical analysis and complexity.
$\bullet$ Stochastic analysis.
Selected Publications of Prof. Dr. Steffen Dereich
$\bullet$ S. Dereich, C. Mailler, and P. Mörters. Nonextensive condensation in reinforced branching processes. Ann. Appl. Probab., 27(4):2539–2568, 2017.
$\bullet$ S. Dereich, L. Döring, and A. E. Kyprianou. Real self-similar processes started from the origin. Ann. Probab., 45(3):1952–2003, 2017.
$\bullet$ S. Dereich and S. Li. Multilevel Monte Carlo for Lévy-driven {SDE}s: central limit theorems for adaptive Euler schemes. Ann. Appl. Probab., 26(1):136–185, 2016.
$\bullet$ S. Dereich. Preferential attachment with fitness: unfolding the condensate. Electron. J. Probab., 21:no 3, 38 pp., 2016.
$\bullet$ S. Dereich and P. Mörters. Random networks with sublinear preferential attachment: the giant component. Ann. Probab., 41(1):329–384, 2013.
$\bullet$ S. Dereich. Multilevel Monte Carlo algorithms for Lévy-driven {SDE}s with Gaussian correction. Ann. Appl. Probab., 21(1):283–311, 2011.
$\bullet$ S. Dereich. Rough paths analysis of general Banach space-valued Wiener processes. J. Funct. Anal., 258(9):2910–2936, 2010.
$\bullet$ S. Dereich and P. Mörters. Random networks with sublinear preferential attachment: degree evolutions. Electron. J. Probab., 14:no. 43, 1222–1267, 2009.
$\bullet$ F. Aurzada and S. Dereich. Small deviations of general Lévy processes. Ann. Probab., 37(5):2066–2092, 2009.
$\bullet$ S. Ankirchner, S. Dereich, and P. Imkeller. The Shannon information of filtrations and the additional logarithmic utility of insiders. Ann. Probab., 34(2):743–778, 2006.
Current Cluster Publications of Prof. Dr. Steffen Dereich
$\bullet $ Steffen Dereich, Robin Graeber, and Arnulf Jentzen. Non-convergence of Adam and other adaptive stochastic gradient descent optimization methods for non-vanishing learning rates. arXiv e-prints, July 2024. arXiv:2407.08100.
$\bullet $ Steffen Dereich and Arnulf Jentzen. Convergence rates for the Adam optimizer. arXiv e-prints, July 2024. arXiv:2407.21078.
$\bullet $ Steffen Dereich, Arnulf Jentzen, and Adrian Riekert. Learning rate adaptive stochastic gradient descent optimization methods: numerical simulations for deep learning methods for partial differential equations and convergence analyses. arXiv e-prints, June 2024. arXiv:2406.14340.
$\bullet $ Steffen Dereich and Sebastian Kassing. Convergence of stochastic gradient descent schemes for Łojasiewicz-landscapes. Journal of Machine Learning, 3(3):245–281, June 2024. doi:10.4208/jml.240109.
$\bullet $ Steffen Dereich and Sebastian Kassing. On the existence of optimal shallow feedforward networks with ReLU activation. Journal of Machine Learning, 3(1):1–22, January 2024. doi:10.4208/jml.230903.
$\bullet $ Steffen Dereich and Sebastian Kassing. On the existence of optimal shallow feedforward networks with ReLU activation. arXiv e-prints, March 2023. arXiv:2303.03950.
$\bullet $ Steffen Dereich, Arnulf Jentzen, and Sebastian Kassing. On the existence of minimizers in shallow residual ReLU neural network optimization landscapes. arXiv e-prints, February 2023. arXiv:2302.14690.
$\bullet $ Steffen Dereich and Sebastian Kassing. Central limit theorems for stochastic gradient descent with averaging for stable manifolds. Electronic Journal of Probability, 28:1–48, January 2023. doi:10.1214/23-EJP947.
$\bullet $ Steffen Dereich and Sebastian Kassing. Cooling down stochastic differential equations: Almost sure convergence. Stoch. Process. their Appl., 152:289–311, October 2022. doi:10.1016/j.spa.2022.06.020.
$\bullet $ Steffen Dereich and Sebastian Kassing. On minimal representations of shallow ReLU networks. Neural Networks, 148:121–128, April 2022. doi:10.1016/j.neunet.2022.01.006.
$\bullet $ Steffen Dereich and Martin Maiwald. Quasi-processes for branching Markov chains. arXiv e-prints, July 2021. arXiv:2107.06654.
$\bullet $ Steffen Dereich and Sebastian Kassing. Convergence of stochastic gradient descent schemes for Lojasiewicz-landscapes. arXiv e-prints, February 2021. arXiv:2102.09385.
$\bullet $ Steffen Dereich. General multilevel adaptations for stochastic approximation algorithms II: CLTs. Stochastic Process. Appl., 132:226–260, February 2021. doi:10.1016/j.spa.2020.11.001.
$\bullet $ Steffen Dereich and Sebastian Kassing. Central limit theorems for stochastic gradient descent with averaging for stable manifolds. arXiv e-prints, December 2019. arXiv:1912.09187.
$\bullet $ Steffen Dereich. The rank-one and the preferential attachment paradigm. In Network Science, pages 43–58. November 2019. doi:10.1007/978-3-030-26814-5_4.
$\bullet $ Steffen Dereich and Thomas Müller-Gronbach. General multilevel adaptations for stochastic approximation algorithms of Robbins–Monro and Polyak–Ruppert type. Numer. Math., 142(2):279–328, June 2019. doi:10.1007/s00211-019-01024-y.