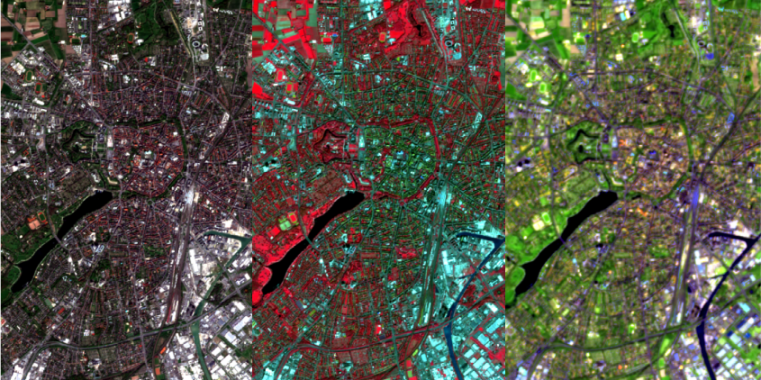
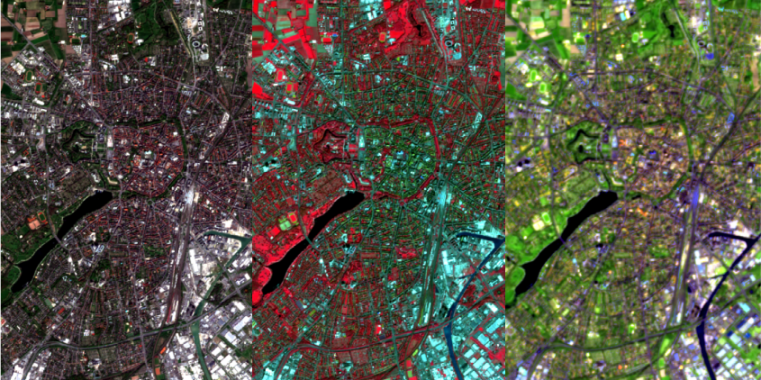
About us
The Research Group of "Remote Sensing and Spatial Modelling" is part of the Institute of Landscape Ecology (ILOEK) [en] of the University of Münster. We study and teach the acquisition and analysis of spatio-temporal environmental dynamics in a board spectrum of landscape-ecological topics. We combine multi-scale remote sensing data with methods of spatial modelling in order to obtain continuous spatio-temporal information from limited ecological field samples.
The complexity of environmental systems requires the use of modelling strategies that take complex relationships into account. For this reason, we focus on the application of machine learning methods. In addition to their application for research questions in the context of landscape ecology, we also develop new modelling strategies for spatial and spatio-temporal data. Thus, the research group is at the interface between Geoinformatics and Landscape Ecology [en] and has the aim to contribute to an increase in knowledge in ecosystem research via drone data acquisition, satellite data processing, modelling and simulation.
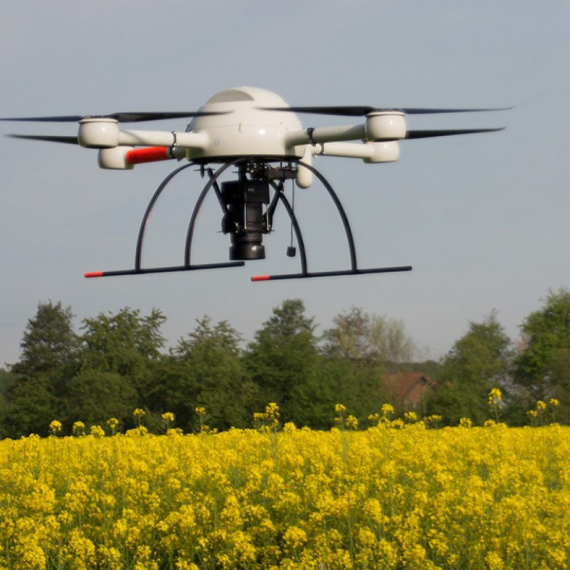
We are looking for a new team member!
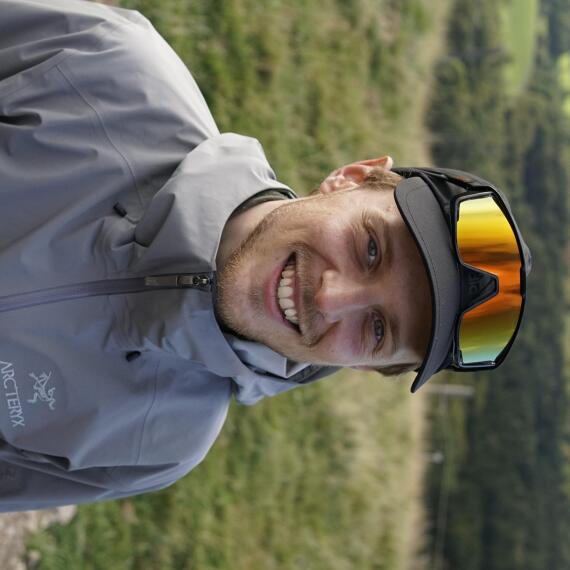
Fabian wins the EGU OSPP Award

Workshop: Agent-based modelling in ecology
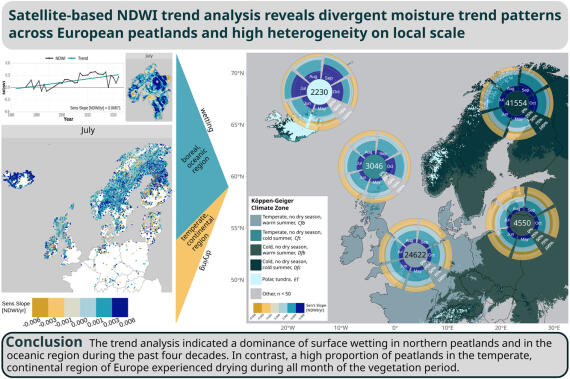
New study reveals significant moisture changes in European peatlands
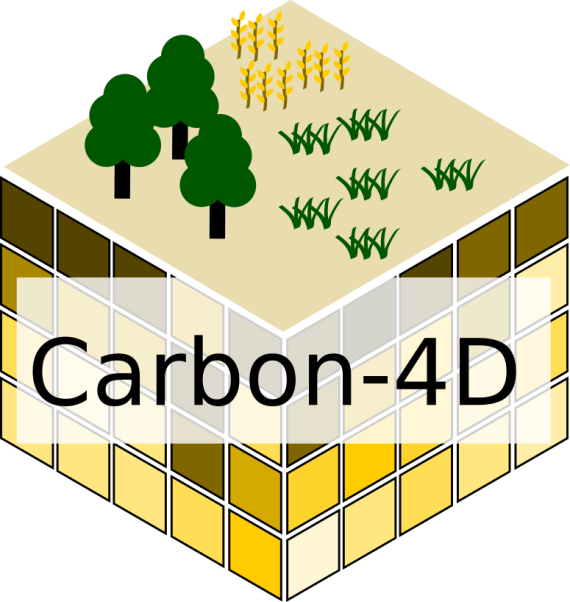
Two new publications in the project Carbon4D

Field survey in Bagno Kusowo
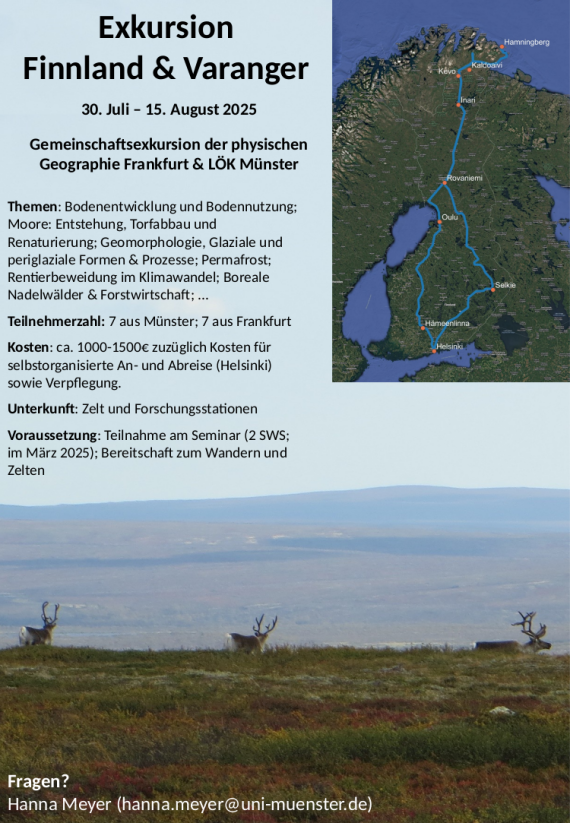
Finland excursion 2025

Welcome, Jakub!
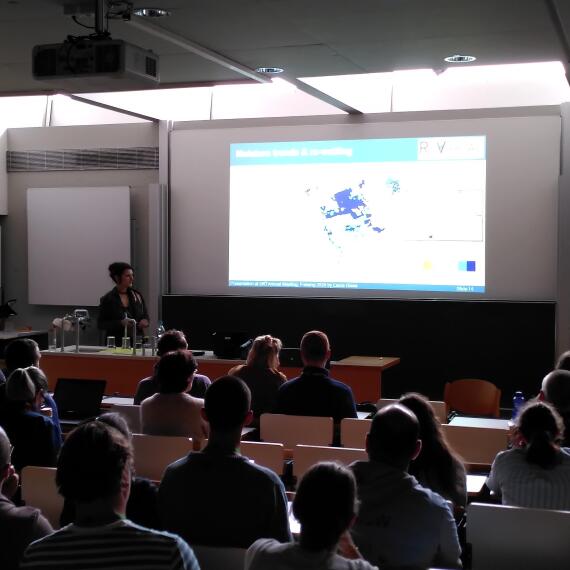
Our Group at the GFÖ Conference in Freising
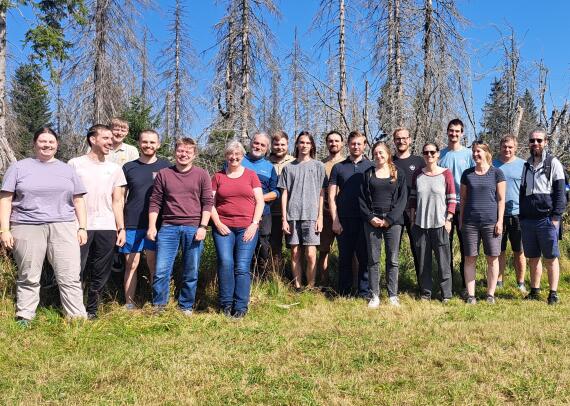
Earth Observation Summer School in the Harz mountains

Impressive Poster Presentations in "Introduction to Remote Sensing"
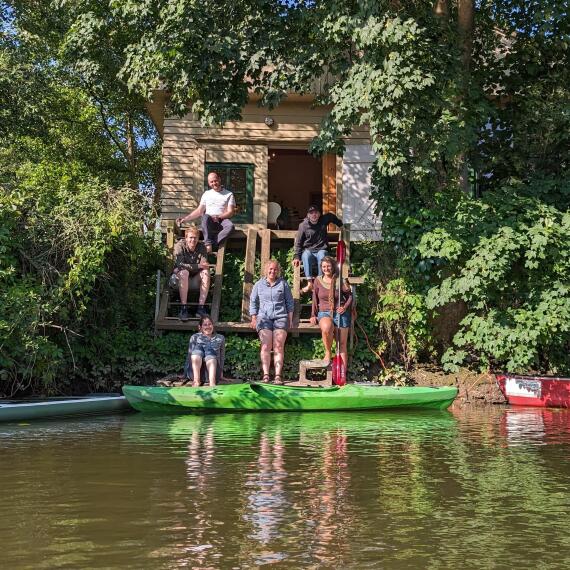
Working group day 2024
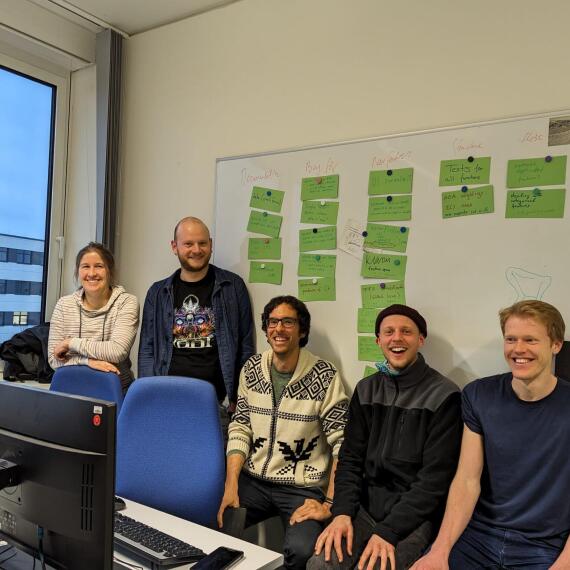
CAST developer week
More News...
Older news can be found in the archive [en] of the Remote Sensing and Spatial Modelling Research Group.