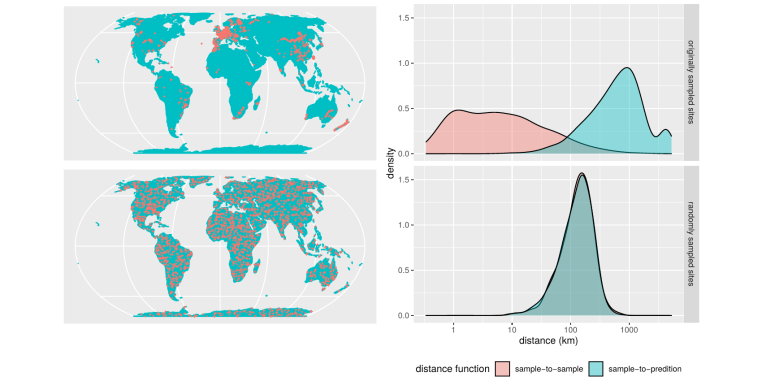
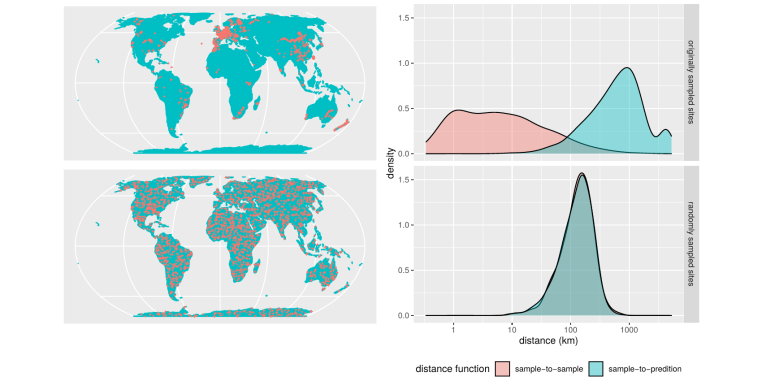
Uebersat: Spatio-temporal transferability of satellite-based AI-models
© BMWi Description
AI methods are increasingly used in the context of satellite-based earth observation to generate spatiotemporal environmental information, for example for monitoring land use, biodiversity patterns or effects of climate change. AI models are usually trained on the basis of local field observations with the aim to make predictions for a larger area and/or a new time for which no reference data are available. However, the transferability of the models to new locations and/or new times is rarely questioned in current AI-applications and models are often applied to make predictions far beyond the geographic location of the training samples. Especially in heterogeneous landscapes the new locations might differ considerably in terms of their environmental characteristics from what has been observed in the training data. This is problematic, since machine learning algorithms can fit very complex relationships, but at the same time are weak at extrapolation. Predictions for new locations/times that differ in their characteristics from the training data must therefore be considered very uncertain, which calls for a method to assess the area of applicability of AI-models.
In this project new methods for the analysis and improvement of the transferability of satellite-based AI models in space and time will be developed, with the aim to assess and increase the quality of earth observation products through the use of innovative AI techniques. The new methods will be integrated into cloud-based processing chains to optimise data-driven applications and deliver more reliable monitoring results.Information
Project lead: Hanna Meyer, Edzer Pebesma (IfGI)
Team@GEOI: Marvin Ludwig, Jonathan Bahlmann, Hanna Meyer, Edzer Pebesma
Funding: BMWi
Term: 2021 - 2023Publikationen
Peer-Review Artikel
- Ludwig M, Moreno-Martinez A, Hölzel N, Pebesma E, Meyer H. 2023. ‘Assessing and improving the transferability of current global spatial prediction models.’ Global Ecology and Biogeography 00: 1–13. doi: https://doi.org/10.1111/geb.13635.
- Meyer H, Pebesma E. 2022. ‘Machine learning-based global maps of ecological variables and the challenge of assessing them.’ Nature Communications 13. doi: 10.1038/s41467-022-29838-9.
- Mila C, Mateu J, Pebesma E, Meyer H. 2022. ‘Nearest neighbour distance matching leave-one-out cross-validation for map validation.’ Methods in Ecology and Evolution n/a. doi: 10.1111/2041-210X.13851.
- Meyer H, Pebesma E (2021): Predicting into unknown space? Estimating the area of applicability of spatial prediction models. Methods in Ecology and Evolution 2021. doi: 10.1111/2041-210X.13650.
Konferenzartikel
- Ludwig M, Bahlmann J, Pebesma E, Meyer H. 2022. ‘Developing Transferable Spatial Prediction Models: a Case Study of Satellite Based Landcover Mapping.’ Contributed to the ISPRS, Nice. doi: 10.5194/isprs-archives-XLIII-B3-2022-135-2022.
- Meyer H, Pebesma E. 2021. ‘Estimating the Area of Applicability of Remote Sensing-Based Machine Learning Models with Limited Training Data.’ Contributed to the IEEE International Geoscience and Remote Sensing Symposium IGARSS, Brussels, Belgium. doi: 10.1109/IGARSS47720.2021.9553999.
Abschlussarbeiten
Thalis Goldschmidt: Development of an interactive web tool to improve the understanding of spatial prediction models and their quality assessment. B.Sc. Geoinformatik.
Lucas Casuccio: Optimizing the allocation of field samples for remote sensing and machine learning-based crop classification models by assessing the area of applicability. M.Sc. Geospatial technologies.
Vinotha Kumar: Development of a Transferable Machine Learning Model for Crop Type Mapping. M.Sc. Geoinformatik.