

Machine Learning for Movement Science
The application of AI methods in the field of human motion analysis has increased significantly in recent years. Machine learning can be applied both as a tool to provide us extra insight into data we have, or to improve our capturing of these data. For example, machine learning has been applied very successfully in the field of human motion analysis and measurement, where they allow us to determine the 3D motion of a human with only one (depth-sensing) camera combined with body shell recognition.
Furthermore, biologically inspired machine learning types - the artificial neural networks - can be used to increase our understanding of how biological neural networks function. By allowing us to manipulate the interconnections, learning rules and network architectures and comparing these findings to recordings of biological neural networks, we can generate hypotheses for how the biological networks function.
Courses
In the ‘Machine Learning for Movement Science’ module, both these aspects will be covered in two separate courses: Recurrent Neural Networks & Motor control and Machine Learning Applications in Movement Science. We aim to offer interesting courses to both informatics students interested in moving into the field of movement science, as well as movement scientists wanting to increase their skill set by learning machine learning (and, of course, to anyone else that is interested in combining these fields!).
In the first half of the courses, you will work your way through several content blocks (with texts, videos, practice exercises, etc.) to get an overview of the field and to have reference material for the second part of the course. There, you will apply what you have learned so far and work on a mini-project for a more hands-on learning experience.
Both courses can be followed fully online, but (optional) support classes will be scheduled for those who appreciate face-to-face contact. Furthermore, it is both possible to audit the course (i.e. check out the course contents at your own pace, any time you want) or to follow the credit point tract (this requires handing in assignments and a final project at the end of the course and can only be done within the semester).
Recurrent Neural Networks & Motor control
In this course, you will learn how you can use a subtype of artificial neural networks, namely recurrent neural networks (RNNs), to learn more about motor control. Amongst others, you will receive an introduction to motor control and RNNs, learn about network architecture choices, the biological plausibility of learning algorithms and we will take a closer look at some studies where researchers have applied RNNs to explore motor control. In this course, we are interested in how our models work, as we use them as a proxy for how biological networks work.
The course is held in winter term 2024/2025. You can find the course in Learnweb. The enrolment key is: Recurrence24. If you have any questions in advance, email mdegraaf@uni-muenster.de.
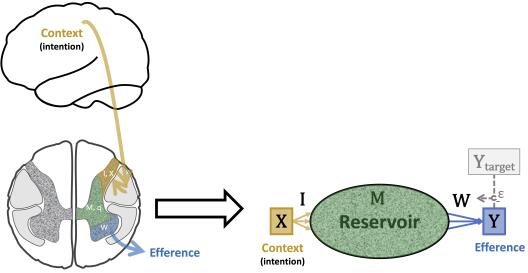
Machine Learning Applications in Movement Science
In this course, we’re not much interested in the design and functioning of the machine learning models themselves, but we view them as tools in our scientific toolbox. We will discuss several ways in which machine learning models can be used in the field of movement sciences, such as markerless motion capture, gait event detection, injury/performance prediction, prosthesis control and more.
This course is offered in the summer term.
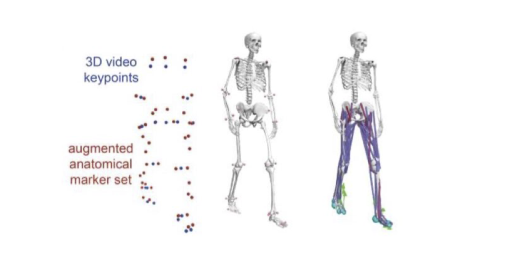